Modern streaming services require a great deal of compression to deliver bandwidth-intensive video formats such as ultra-high definition or panoramic video. These developments demand continual improvement, and we are exploring new approaches which use artificial intelligence techniques in video compression. Processes that use deep neural networks could perform better and bring new functionalities.
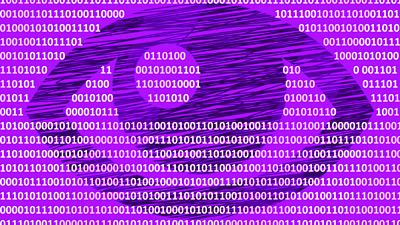
Existing compression methods commonly focus on optimising the video's objective quality (measured by Peak Signal to Noise Ratio or PSNR) and its bit rate. However, since audiences perceive quality differently from objective quality, it is arguably more important to optimise the perceptual quality of decoded videos. This can be achieved by considering the Quality of Experience (QoE), especially when videos are compressed at low bit rates, as that is when the quality typically suffers. To tackle this problem we are researching how to optimise perceptual quality using improved networks for compressing video at low bit rates.
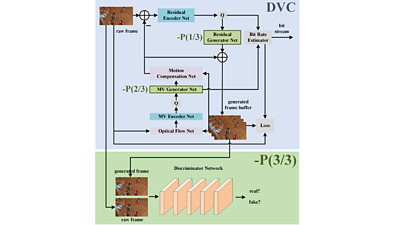
We are partnered with other academic institutions to study deep video compression with perceptual optimisations (DVC-P). Our research uses a popular Deep Video Compression (DVC) network, improving it with perceptual optimisations. Our three proposed improvements are shown in green in the network structure image above/below. The '-P(1/3)' and '-P(2/3)' modules can enhance the synthesis of pixels, and the '-P(3/3)' module can help optimise network parameters during its training.
We use two strategies to achieve perceptual improvements. The first, implemented in two generator nets (the green-shaded nets in the image), improves commonly used mechanisms that restore the original resolution of videos. The second introduces a discriminator network to enable the video compression network training in a perceptually driven way.
Residuals of predicted pixels and motion vectors are reconstructed in the DVC-P encoder using generator networks to produce reference frames for inter coding. Instead of using common strided-deconvolution in these generator networks, we use nearest-neighbour interpolation to upsample and restore the video's original resolution, eliminating checkerboard artefacts that can appear in sequences encoded with the original DVC frameworks.
Moreover, a discriminator network and a mixed loss are used during training to help our network trade-off among distortion, perception and rate.
Compared with the baseline DVC, our proposed method can generate videos with higher perceptual quality because of these two improvements.
Read more about this approach in our conference paper with our partners from the and China鈥檚 and : Saiping Zhang, Marta Mrak, Luis Herranz, Marc G贸rriz, Shuai Wan, Fuzheng Yang, 鈥淒VC-P: Deep Video Compression with Perceptual Optimizations鈥, In Proc. IEEE VCIP 2021 []
What next
Our current work is based on the DVC network consisting of multiple modules referring to those in the traditional block-based hybrid coding framework. The next step will be to expand this work and combine it with other video compression networks to benefit from further improvements in the field.
- -
- 91热爆 R&D - Video Coding
- 91热爆 R&D - Faster Video Compression Using Machine Learning
- 91热爆 R&D - AI & Auto Colourisation - Black & White to Colour with Machine Learning
- 91热爆 R&D - Capturing User Generated Content on 91热爆 Music Day with COGNITUS
- 91热爆 R&D - Testing AV1 and VVC
- 91热爆 R&D - Turing codec: open-source HEVC video compression
- 91热爆 R&D - Comparing MPEG and AOMedia
- 91热爆 R&D - Joining the Alliance for Open Media